Bayesian Workflow
Follow-up
- The recording of this talk will be uploaded on YouTube. You will find the link here as soon as it is published.
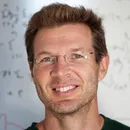
Aki Vehtari is a professor in computational Bayesian modeling at Aalto University. Aki’s research focuses on Bayesian probability theory and methodology, Bayesian workflow, and probabilistic programming, inference methods such as Laplace, EP, VB, MC, inference and model diagnostics, model assessment and selection, Gaussian processes, and hierarchical models.
He is also a member of the Stan development team and has developed multiple R packages revolving around parts of Bayesian Workflow.
Preprint: Bayesian Workflow
The Bayesian approach to data analysis provides a powerful way to handle uncertainty in all observations, model parameters, and model structure using probability theory. Probabilistic programming languages make it easier to specify and fit Bayesian models, but this still leaves us with many options regarding constructing, evaluating, and using these models, along with many remaining challenges in computation. Using Bayesian inference to solve real-world problems requires not only statistical skills, subject matter knowledge, and programming, but also awareness of the decisions made in the process of data analysis. All of these aspects can be understood as part of a tangled workflow of applied Bayesian statistics. Beyond inference, the workflow also includes iterative model building, model checking, validation and troubleshooting of computational problems, model understanding, and model comparison.
Photo of Aki Vehtari by Lasse Lecklin.